Network-Based Approaches in Bioinformatics and Cheminformatics: Leveraging IT for Insights
DOI:
https://doi.org/10.18034/abcjar.v7i2.743Keywords:
Bioinformatics, Computational Biology, Chemogenomics, Biological Networks, Data Integration, Machine LearningAbstract
Network-based approaches in bioinformatics and cheminformatics use computational methods and IT to investigate complicated biological and chemical systems. This review examines network-based analyses' fundamentals, sophisticated techniques, and different applications in these disciplines. The study will investigate novel methods for integrating heterogeneous data sources to build comprehensive biological and chemical networks, apply advanced network analysis techniques to reveal hidden relationships and functional modules within these networks, and assess the efficacy of network-based approaches in elucidating complex biological processes and accelerating drug discovery Network-based approach literature. Secondary data sources are reviewed, focusing on secondary data-based review papers. Significant findings show how network-based methods affect biological processes, disease mechanisms, and medication development. For network-based bioinformatics and cheminformatics to succeed, policymakers must invest in data infrastructure, standardized data formats, and interdisciplinary collaboration. Network-based techniques can use IT to understand biological and chemical systems, shaping biomedical research and precision medicine.
Downloads
References
Anumandla, S. K. R. (2018). AI-enabled Decision Support Systems and Reciprocal Symmetry: Empowering Managers for Better Business Outcomes. International Journal of Reciprocal Symmetry and Theoretical Physics, 5, 33-41. https://upright.pub/index.php/ijrstp/article/view/129
Charleston, P., Angelova, M., Efremova, M., Gallasch, R., Hackl, H. (2012). Bioinformatics for Cancer Immunology and Immunotherapy. Cancer Immunology, Immunotherapy, 61(11), 1885-903. https://doi.org/10.1007/s00262-012-1354-x DOI: https://doi.org/10.1007/s00262-012-1354-x
Ferguson, L. B., Harris, R. A., Mayfield, R. D. (2018). From Gene Networks to Drugs: Systems Pharmacology Approaches for AUD. Psychopharmacology, 235(6), 1635-1662. https://doi.org/10.1007/s00213-018-4855-2 DOI: https://doi.org/10.1007/s00213-018-4855-2
Gu, J., Chen, L., Yuan, G., Xu, X. (2013). A Drug-Target Network-Based Approach to Evaluate the Efficacy of Medicinal Plants for Type II Diabetes Mellitus. Evidence - Based Complementary and Alternative Medicine, 2013. https://doi.org/10.1155/2013/203614 DOI: https://doi.org/10.1155/2013/203614
Hao, M., Bryant, S. H., Wang, Y. (2018). A New Chemoinformatics Approach with Improved Strategies for Effective Predictions of Potential Drugs. Journal of Cheminformatics, 10(1), 1-9. https://doi.org/10.1186/s13321-018-0303-x DOI: https://doi.org/10.1186/s13321-018-0303-x
Hsin, K-Y., Ghosh, S., Kitano, H. (2013). Combining Machine Learning Systems and Multiple Docking Simulation Packages to Improve Docking Prediction Reliability for Network Pharmacology. PLoS One, 8(12), e83922. https://doi.org/10.1371/journal.pone.0083922 DOI: https://doi.org/10.1371/journal.pone.0083922
Karatzas, E., Bourdakou, M. M., Kolios, G., Spyrou, G. M. (2017). Drug Repurposing in Idiopathic Pulmonary Fibrosis Filtered by a Bioinformatics-derived Composite Score. Scientific Reports (Nature Publisher Group), 7, 1-18. https://doi.org/10.1038/s41598-017-12849-8 DOI: https://doi.org/10.1038/s41598-017-12849-8
Khair, M. A. (2018). Security-Centric Software Development: Integrating Secure Coding Practices into the Software Development Lifecycle. Technology & Management Review, 3, 12-26. https://upright.pub/index.php/tmr/article/view/124
Ligeti, B., Pénzváltó, Z., Vera, R., Győrffy, B., Pongor, S. (2015). A Network-Based Target Overlap Score for Characterizing Drug Combinations: High Correlation with Cancer Clinical Trial Results. PLoS One, 10(6), e0129267. https://doi.org/10.1371/journal.pone.0129267 DOI: https://doi.org/10.1371/journal.pone.0129267
Mullangi, K. (2017). Enhancing Financial Performance through AI-driven Predictive Analytics and Reciprocal Symmetry. Asian Accounting and Auditing Advancement, 8(1), 57–66. https://4ajournal.com/article/view/89
Sandu, A. K., Surarapu, P., Khair, M. A., & Mahadasa, R. (2018). Massive MIMO: Revolutionizing Wireless Communication through Massive Antenna Arrays and Beamforming. International Journal of Reciprocal Symmetry and Theoretical Physics, 5, 22-32. https://upright.pub/index.php/ijrstp/article/view/125
Shajahan, M. A. (2018). Fault Tolerance and Reliability in AUTOSAR Stack Development: Redundancy and Error Handling Strategies. Technology & Management Review, 3, 27-45. https://upright.pub/index.php/tmr/article/view/126
Tejani, J. G. (2017). Thermoplastic Elastomers: Emerging Trends and Applications in Rubber Manufacturing. Global Disclosure of Economics and Business, 6(2), 133-144. https://doi.org/10.18034/gdeb.v6i2.737 DOI: https://doi.org/10.18034/gdeb.v6i2.737
Xue, M., Zhang, S., Cai, C., Yu, X., Shan, L. (2013). Predicting the Drug Safety for Traditional Chinese Medicine through a Comparative Analysis of Withdrawn Drugs Using Pharmacological Network. Evidence - Based Complementary and Alternative Medicine, 2013. https://doi.org/10.1155/2013/256782 DOI: https://doi.org/10.1155/2013/256782
Ying, D., Patel, B., & Dhameliya, N. (2017). Managing Digital Transformation: The Role of Artificial Intelligence and Reciprocal Symmetry in Business. ABC Research Alert, 5(3), 67–77. https://doi.org/10.18034/ra.v5i3.659 DOI: https://doi.org/10.18034/ra.v5i3.659
Downloads
Published
Issue
Section
License
Copyright (c) 2018 Rajani Pydipalli

This work is licensed under a Creative Commons Attribution-NonCommercial 4.0 International License.
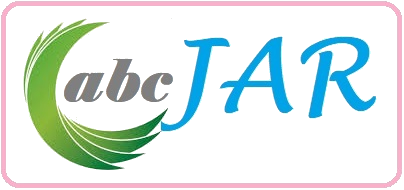