Speech Emotion Recognition Using Deep Learning Techniques
DOI:
https://doi.org/10.18034/abcjar.v5i2.550Keywords:
Deep learning, LSTM, emotional speech database, speech emotion recognitionAbstract
The developments in neural systems and the high demand requirement for exact and close actual Speech Emotion Recognition in human-computer interfaces mark it compulsory to liken existing methods and datasets in speech emotion detection to accomplish practicable clarifications and a securer comprehension of this unrestricted issue. The present investigation assessed deep learning methods for speech emotion detection with accessible datasets, tracked by predictable machine learning methods for SER. Finally, we present-day a multi-aspect assessment between concrete neural network methods in SER. The objective of this investigation is to deliver a review of the area of distinct SER.
Downloads
References
Albert, M. (1996). Pleasure-arousal-dominance: A general framework for describing and measuring individual differences in Temperament. Curr. Psychol., 14, 261–292.
Amer, M.; Siddiquie, B.; Richey, C. and Divakaran, A. (2014). Emotion Detection in Speech Using Deep Networks. In Proceedings of the 2014 IEEE International Conference on Acoustics, Speech and Signal Processing (ICASSP), Florence, Italy, 4–9 May 2014.
Amir, N.; Kerret, O. and Karlinski, D. (2001). Classifying emotions in speech: A comparison of methods. In Proceedings of the Seventh European Conference on Speech Communication and Technology, Aalborg, Denmark, 3–7 September 2001.
Balti, H. and Elmaghraby, A.S. (2013). Speech emotion detection using time dependent self-organizing maps. In Proceedings of the IEEE International Symposium on Signal Processing and Information Technology, Athens, Greece, 12–15 December 2013.
Balti, H. and Elmaghraby, A.S. (2014). Emotion analysis from speech using temporal contextual trajectories. In Proceedings of the IEEE Symposium on Computers and Communications (ISCC), Funchal, Portugal, 23–26 June 2014.
Booth, P.A. (1989). An Introduction to Human-Computer Interaction; Psychology Press: Hove, UK.
Cambria, E.; Hussain, A.; Havasi, C. and Eckl, C. (2010). Sentic computing: Exploitation of common sense for the development of emotion sensitive systems. In Development of Multimodal Interfaces: Active Listening and Synchrony; Springer: Berlin/Heidelberg, Germany, pp. 148–156.
Chavhan, Y.; Dhore, M. and Pallavi, Y. (2010). Speech Emotion Recognition Using Support Vector Machines. Int. J. Comput. Appl., 1, 86–91.
Erden, M. and Arslan, L.M. (2011). Automatic detection of anger in human-human call center dialogs. In Proceedings of the Twelfth Annual Conference of the International Speech Communication Association, Florence, Italy, 27–31 August 2011.
France, D.J.; Shiavi, R.G.; Silverman, S.; Silverman, M. and Wilkes, D.M. (2000). Acoustical Properties of Speech as Indicators of Depression and Suicidal Risk. IEEE Trans. Biomed. Eng., 47, 829–837.
Gobl, C. and Chasaide, A.N. (2003). The role of voice quality in communicating emotion, mood and attitude. Speech Commun., 40, 189–212.
Grimm, M.; Kroschel, K. and Narayanan, S. (2008). The Vera am Mittag German audio-visual emotional speech database. In Proceedings of the IEEE International Conference on Multimedia and Expo, Hannover, Germany, 23–26 June 2008.
Han, K.; Yu, D. and Tashev, I. (2014). Speech Emotion Recognition Using Deep Neural Network and Extreme Learning Machine. In Proceedings of the Fifteenth Annual Conference of the International Speech Communication Association, Singapore, 14–18 September 2014.
Hansen, J.H. and Cairns, D.A. (1995). ICARUS: Source generator based real-time recognition of speech in noisy stressful and Lombard effect environments. Speech Commun., 16, 391–422.
Harper, E.R.; Rodden, T.; Rogers, Y. and Sellen, A. (2008). Being Human: Human-Computer Interaction in the Year 2020; Microsoft Research: Redmond, WA, USA; ISBN 0955476119.
Hassan, A. and Damper, R.I. (2010). Multi-class and hierarchical SVMs for emotion recognition. In Proceedings of the INTERSPEECH 2010, Makuhari, Japan, 26–30 September 2010; pp. 2354–2357.
Kaushik, L.; Sangwan, A. and Hansen, J.H.L. (2013). Sentiment extraction from natural audio streams. In Proceedings of the 2013 IEEE International Conference on Acoustics, Speech and Signal Processing, Vancouver, BC, Canada, 26–31 May 2013.
Lee, C.M. and Narayanan, S. (2005). Toward detecting emotions in spoken dialogs. IEEE Trans. Speech Audio Process. 13, 293–303.
Lin, Y.L. and Wei, G. (2005). Speech emotion recognition based on HMM and SVM. In Proceedings of the 2005 International Conference on Machine Learning and Cybernetics, Guangzhou, China, 18–21 August 2005; Volume 8, pp. 4898–4901.
Lugger, M. and Yang, B. (2007). The relevance of voice quality features in speaker independent emotion recognition. ICASSP, 4, 17–20.
Movva, L., Kurra, C., Koteswara Rao, G., Battula, R. B., Sridhar, M., & Harish, P. (2012). Underwater Acoustic Sensor Networks: A Survey on MAC and Routing Protocols. International Journal of Computer Technology and Applications, 3(3).
Nakatsu, R.; Nicholson, J. and Tosa, N. (2000). Emotion recognition and its application to computer agents with spontaneous interactive capabilities. Knowl.-Based Syst., 13, 497–504.
Neogy, T. K., & Paruchuri, H. (2014). Machine Learning as a New Search Engine Interface: An Overview. Engineering International, 2(2), 103-112. https://doi.org/10.18034/ei.v2i2.539
Nicholson, J.; Takahashi, K. and Nakatsu, R. (1999). Emotion Recognition in Speech Using Neural Networks. In Proceedings of the 6th International Conference on Neural Information Processing (ICONIP ’99), Perth, Australia, 16–20 November 1999.
Nwe, T.L.; Foo, S.W. and De Silva, L.C. (2003). Speech emotion recognition using hidden Markov models. Speech Commun., 41, 603–623.
Paruchuri, H. (2015). Application of Artificial Neural Network to ANPR: An Overview. ABC Journal of Advanced Research, 4(2), 143-152. https://doi.org/10.18034/abcjar.v4i2.549
Patil, K.J.; Zope, P.H. and Suralkar, S.R. (2012). Emotion Detection From Speech Using Mfcc and Gmm. Int. J. Eng. Res. Technol. (IJERT), 1, 9.
Petrushin, V. (2000). Emotion in Speech: Recognition and Application to Call Centers. Artif. Neural Netw. Eng. 2000, 710, 22.
Philippou-Hübner, D.; Vlasenko, B.; Grosser, T. and Wendemuth, A. (2010). Determining optimal features for emotion recognition from speech by applying an evolutionary algorithm. In Proceedings of the Eleventh Annual Conference of the International Speech Communication Association, Chiba, Japan, 26–30 September 2010; pp. 2358–2361.
Schüller, B. and Rigoll, G. (2006). Timing levels in segment-based speech emotion recognition. In Proceedings of the Ninth International Conference on Spoken Language Processing, Pittsburgh, PA, USA, 17–21 September 2006; pp. 17–21.
Schüller, B.; Rigoll, G. and Lang, M. (2004). Speech emotion recognition combining acoustic features and linguistic information in a hybrid support vector machine-belief network architecture. In Proceedings of the IEEE International Conference on Acoustics, Speech, and Signal Processing, Montreal, QC, Canada, 17–21 May 2004.
Sepp Hochreiter, J.S. (1997). Long Short-Term Memory. Neural Comput., 9, 1735–1780.
Shaw, A.; Vardhan, R.K. and Saxena, S. (2016). Emotion Recognition and Classification in Speech using Artificial Neural Networks. Int. J. Comput. Appl., 145, 5–9.
Song, P.; Jin, Y.; Zhao, L. and Xin, M. (2014). Speech Emotion Recognition Using Transfer Learning. IEICE Trans. Inf. Syst., 97, 2530–2532.
Stuhlsatz, A.; Meyer, C.; Eyben, F.; Zielke, T.; Meier, H.G. and Schüller, B. (2011). Deep neural networks for acoustic emotion recognition: Raising the benchmarks. In Proceedings of the 2011 IEEE international conference on acoustics, speech and signal processing (ICASSP), Prague, Czech Republic, 22–27 May 2011.
Trigeorgis, G.; Ringeval, F.; Brueckner, R.; Marchi, E.; Nicolaou, M.A.; Schüller, B. and Zafeiriou, S. (2016). Adieu Features? End-To-End Speech Emotion Recognition Using A Deep Convolutional Recurrent Network. In Proceedings of the IEEE International Conference on Acoustics, Speech and Signal Processing (ICASSP), Shanghai, China, 20–25 March 2016.
Truong, K.P.; van Leeuwen, D.A. and de Jong, F.M.G. (2012). Speech-based recognition of self-reported and observed emotion in a dimensional space. Speech Commun., 54, 1049–1063.
Ujwala, D., Ram Kiran, D. S., Jyothi, B., Fathima, S. S., Paruchuri, H., Koushik, Y. M. S. R. (2012). A Parametric Study on Impedance Matching of A CPW Fed T-shaped UWB Antenna. International Journal of Soft Computing and Engineering, 2(2), 433-436.
Vadlamudi, S. (2015). Enabling Trustworthiness in Artificial Intelligence - A Detailed Discussion. Engineering International, 3(2), 105-114. https://doi.org/10.18034/ei.v3i2.519
Vadlamudi, S. (2016). What Impact does Internet of Things have on Project Management in Project based Firms?. Asian Business Review, 6(3), 179-186. https://doi.org/10.18034/abr.v6i3.520
Vlasenko, B.; Prylipko, D.; Philippou-Hübner, D. and Wendemuth, A. (2011). Vowels formants analysis allows straightforward detection of high arousal acted and spontaneous emotions. In Proceedings of the Twelfth Annual Conference of the International Speech Communication Association, Florence, Italy, 27–31 August 2011; pp. 1577–1580.
Williams, C.E. and Stevens, K.N. (1972). Emotions and Speech: Some Acoustical Correlates. J. Acoust. Soc. Am., 52, 1238–1250.
Wöllmer, M.; Kaiser, M.; Eyben, F.; Schüller, B. and Rigoll, G. (2013). LSTM-Modeling of continuous emotions in an audiovisual affect recognition framework. Image Vis. Comput., 31, 153–163.
--0--
Downloads
Published
Issue
Section
License
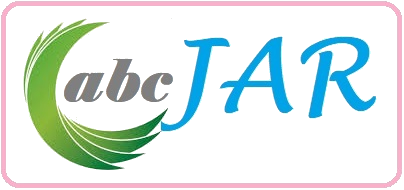