Machine Learning-Enhanced Beamforming with Smart Antennas in Wireless Networks
DOI:
https://doi.org/10.18034/abcjar.v10i2.770Keywords:
Machine Learning, Beamforming, Smart Antennas, Wireless Networks, Signal Processing, Adaptive Algorithms, Channel Estimation, Reinforcement LearningAbstract
This research integrates machine learning (ML) approaches into beamforming using smart antennas to improve wireless networks. The main goals are to evaluate ML-driven beamforming techniques for enhancing SNR, BER, and throughput while tackling dynamic environments and interference. The study synthesizes simulation and experimental results using secondary data. Significant results show that ML-enhanced beamforming outperforms standard approaches by improving SNR by 15 dB, lowering BER by 30-50%, and decreasing interference. However, sophisticated ML algorithms are computationally demanding and need high-quality training data. Policy implications emphasize the need for effective data governance frameworks to assure data integrity, security, and efficient algorithms that can function within infrastructure restrictions. Stakeholders should collaborate to create standardized methods that optimize the advantages of ML-enhanced beamforming while addressing concerns, opening the door for more intelligent, more adaptable wireless communication systems.
Downloads
References
Allam, A. R. (2020). Integrating Convolutional Neural Networks and Reinforcement Learning for Robotics Autonomy. NEXG AI Review of America, 1(1), 101-118.
Almeida, N. C., Fernandes, M. A. C., Neto, A. D. D. (2015). Beamforming and Power Control in Sensor Arrays Using Reinforcement Learning. Sensors, 15(3), 6668-6687. https://doi.org/10.3390/s150306668 DOI: https://doi.org/10.3390/s150306668
Boinapalli, N. R. (2020). Digital Transformation in U.S. Industries: AI as a Catalyst for Sustainable Growth. NEXG AI Review of America, 1(1), 70-84.
Devarapu, K., Rahman, K., Kamisetty, A., & Narsina, D. (2019). MLOps-Driven Solutions for Real-Time Monitoring of Obesity and Its Impact on Heart Disease Risk: Enhancing Predictive Accuracy in Healthcare. International Journal of Reciprocal Symmetry and Theoretical Physics, 6, 43-55. https://upright.pub/index.php/ijrstp/article/view/160
Engmann, F., Katsriku, F. A., Abdulai, J-D., Adu-Manu, K. S., Banaseka, F. K. (2018). Prolonging the Lifetime of Wireless Sensor Networks: A Review of Current Techniques. Wireless Communications & Mobile Computing (Online), 2018. https://doi.org/10.1155/2018/8035065 DOI: https://doi.org/10.1155/2018/8035065
Famoriji, O. J., Zhang, Z., Fadamiro, A., Zakariyya, R., Lin, F. (2018). Planar Array Diagnostic Tool for Millimeter-Wave Wireless Communication Systems. Electronics, 7(12), 383. https://doi.org/10.3390/electronics7120383 DOI: https://doi.org/10.3390/electronics7120383
Gummadi, J. C. S., Narsina, D., Karanam, R. K., Kamisetty, A., Talla, R. R., & Rodriguez, M. (2020). Corporate Governance in the Age of Artificial Intelligence: Balancing Innovation with Ethical Responsibility. Technology & Management Review, 5, 66-79. https://upright.pub/index.php/tmr/article/view/157
Jia-xin, C., Zhong, R., Li, Y. (2019). Antenna Selection for Multiple-input Multiple-output Systems Based on Deep Convolutional Neural Networks. PLoS One, 14(5), e0215672. https://doi.org/10.1371/journal.pone.0215672 DOI: https://doi.org/10.1371/journal.pone.0215672
Karanam, R. K., Natakam, V. M., Boinapalli, N. R., Sridharlakshmi, N. R. B., Allam, A. R., Gade, P. K., Venkata, S. G. N., Kommineni, H. P., & Manikyala, A. (2018). Neural Networks in Algorithmic Trading for Financial Markets. Asian Accounting and Auditing Advancement, 9(1), 115–126. https://4ajournal.com/article/view/95
Kommineni, H. P., Fadziso, T., Gade, P. K., Venkata, S. S. M. G. N., & Manikyala, A. (2020). Quantifying Cybersecurity Investment Returns Using Risk Management Indicators. Asian Accounting and Auditing Advancement, 11(1), 117–128. Retrieved from https://4ajournal.com/article/view/97
Kothapalli, S., Manikyala, A., Kommineni, H. P., Venkata, S. G. N., Gade, P. K., Allam, A. R., Sridharlakshmi, N. R. B., Boinapalli, N. R., Onteddu, A. R., & Kundavaram, R. R. (2019). Code Refactoring Strategies for DevOps: Improving Software Maintainability and Scalability. ABC Research Alert, 7(3), 193–204. https://doi.org/10.18034/ra.v7i3.663 DOI: https://doi.org/10.18034/ra.v7i3.663
Kundavaram, R. R., Rahman, K., Devarapu, K., Narsina, D., Kamisetty, A., Gummadi, J. C. S., Talla, R. R., Onteddu, A. R., & Kothapalli, S. (2018). Predictive Analytics and Generative AI for Optimizing Cervical and Breast Cancer Outcomes: A Data-Centric Approach. ABC Research Alert, 6(3), 214-223. https://doi.org/10.18034/ra.v6i3.672 DOI: https://doi.org/10.18034/ra.v6i3.672
Li, Q., Dai, K., Wang, X., Zhang, Y., Zhang, H. (2019). Low-Complexity Failed Element Diagnosis for Radar-Communication mmWave Antenna Array with Low SNR. Electronics, 8(8), 904. https://doi.org/10.3390/electronics8080904 DOI: https://doi.org/10.3390/electronics8080904
Memon, M. L., Saxena, N., Roy, A., Shin, D. R. (2019). Backscatter Communications: Inception of the Battery-Free Era—A Comprehensive Survey. Electronics, 8(2), 129. https://doi.org/10.3390/electronics8020129 DOI: https://doi.org/10.3390/electronics8020129
Minoli, D., Occhiogrosso, B. (2019). Practical Aspects for the Integration of 5G Networks and IoT Applications in Smart Cities Environments. Wireless Communications & Mobile Computing (Online), 2019. https://doi.org/10.1155/2019/5710834 DOI: https://doi.org/10.1155/2019/5710834
Rodriguez, M., Mohammed, M. A., Mohammed, R., Pasam, P., Karanam, R. K., Vennapusa, S. C. R., & Boinapalli, N. R. (2019). Oracle EBS and Digital Transformation: Aligning Technology with Business Goals. Technology & Management Review, 4, 49-63. https://upright.pub/index.php/tmr/article/view/151
Rodriguez, M., Sridharlakshmi, N. R. B., Boinapalli, N. R., Allam, A. R., & Devarapu, K. (2020). Applying Convolutional Neural Networks for IoT Image Recognition. International Journal of Reciprocal Symmetry and Theoretical Physics, 7, 32-43. https://upright.pub/index.php/ijrstp/article/view/158
Singh, K. D., Rawat, P., Bonnin, J-m. (2014). Cognitive Radio for Vehicular Ad Hoc Networks (CR-VANETs): Approaches and Challenges. EURASIP Journal on Wireless Communications and Networking, 2014, 1-22. https://doi.org/10.1186/1687-1499-2014-49 DOI: https://doi.org/10.1186/1687-1499-2014-49
Sridharlakshmi, N. R. B. (2020). The Impact of Machine Learning on Multilingual Communication and Translation Automation. NEXG AI Review of America, 1(1), 85-100.
Sultan, K., Hazrat, A., Zhang, Z. (2018). Big Data Perspective and Challenges in Next Generation Networks. Future Internet, 10(7). https://doi.org/10.3390/fi10070056 DOI: https://doi.org/10.3390/fi10070056
Thompson, C. R., Talla, R. R., Gummadi, J. C. S., Kamisetty, A (2019). Reinforcement Learning Techniques for Autonomous Robotics. Asian Journal of Applied Science and Engineering, 8(1), 85-96. https://ajase.net/article/view/94 DOI: https://doi.org/10.18034/ajase.v8i1.94
Yıldız, A., Dzakmic, Š., Saleh, M. A. (2019). A Short Survey on Next Generation 5G Wireless Networks. Sustainable Engineering and Innovation, 1(1), 57-66. https://doi.org/10.37868/sei.v1i1.93 DOI: https://doi.org/10.37868/sei.v1i1.93
Downloads
Published
Issue
Section
License
Copyright (c) 2021 Pavan Kumar Gade; Narayana Reddy Bommu Sridharlakshmi; Abhishekar Reddy Allam; Samuel Koehler

This work is licensed under a Creative Commons Attribution-NonCommercial 4.0 International License.
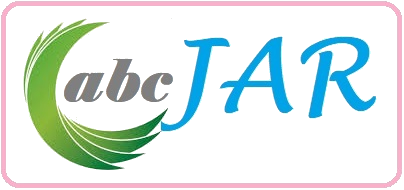