An Approach to Enhance Text Categorization through Shrinkage in a Hierarchy of Modules
DOI:
https://doi.org/10.18034/abcjar.v8i2.562Keywords:
Text Categorization, Shrinkage, Naïve Bayes, Hierarchy of modulesAbstract
Most organizations carried out their activities by design and develop a large volume of programmed documents as an essential element of their external and internal performance. When documents are well-known in a large volume of subject matter classification, the classifications are frequently prepared in order. Newsgroup and yahoo databases are two cases studied. This article indicates that the precision of a naïve Bayes text classifier can be importantly enhanced by taking benefit of a hierarchy of categories. A statistical approach known as shrinkage was adopted that levels variable prediction of a data-sparse child with its blood relation in direction to acquire more vigorous variable predictions. The test results on 3 real-time datasets from Yahoo, UseNet, and shared webpages display enhanced performance with about 29% error reduction over the customarily flat classifier.
Downloads
References
Carlin, B. and Louis, T. (1996). Bayes and Empirical Bayes Methods for Data Analysis. Chapman and Hall.
Cohen, W. W. (1995). Fast effective rule induction, in ‘International Conference on Machine Learning’, pp. 115–123. DOI: https://doi.org/10.1016/B978-1-55860-377-6.50023-2
D’Alessio, S., Murray, K., Schiaffino, R. & Kershenbaum, A. (2000). The effect of using hierarchical classifiers in text categorization, in ‘Proc. of the 6th Int. Conf. “Recherche d’Information Assistee par Ordinateur”’, Paris, FR, pp. 302–313.
Dempster, AP., Laird, NM., and Rubin, DB. (1977). Maximum likelihood from incomplete data via the EM algorithm. Journal of the Royal Statistical Society, Series B, 39:1-38. DOI: https://doi.org/10.1111/j.2517-6161.1977.tb01600.x
Dumais, S. T. & Chen, H. (2000). Hierarchical classification of Web content, in ‘Proc. of the 23rd ACM Int. Conf. on Research and Development in Information Retrieval (SIGIR)’, Athens, GR, pp. 256–263. DOI: https://doi.org/10.1145/345508.345593
EePeng, LIM., Aixin, SUN. and Wee-Keong, NG. (2003). Performance measurement framework for hierarchical text classification. Journal of the American Society for Information Science and Technology (JASIST). 54, (11), 1014-1028. Research Collection School Of Information Systems. Available at: https://ink.library.smu.edu.sg/sis_research/166 DOI: https://doi.org/10.1002/asi.10298
Ganapathy, A. (2016). Speech Emotion Recognition Using Deep Learning Techniques. ABC Journal of Advanced Research, 5(2), 113-122. https://doi.org/10.18034/abcjar.v5i2.550 DOI: https://doi.org/10.18034/abcjar.v5i2.550
Ganapathy, A. (2017). Friendly URLs in the CMS and Power of Global Ranking with Crawlers with Added Security. Engineering International, 5(2), 87-96. https://doi.org/10.18034/ei.v5i2.541 DOI: https://doi.org/10.18034/ei.v5i2.541
Ganapathy, A. (2018). Cascading Cache Layer in Content Management System. Asian Business Review, 8(3), 177-182. https://doi.org/10.18034/abr.v8i3.542 DOI: https://doi.org/10.18034/abr.v8i3.542
Ganapathy, A., & Neogy, T. K. (2017). Artificial Intelligence Price Emulator: A Study on Cryptocurrency. Global Disclosure of Economics and Business, 6(2), 115-122. https://doi.org/10.18034/gdeb.v6i2.558 DOI: https://doi.org/10.18034/gdeb.v6i2.558
James, W. and Stein, C. (1961). Estimation with quadratic loss. In Proceedings of the Fourth Berkeley Symposium on Mathematical Statistics and Probability 1. Pp. 361-379. University of California Press.
Joachims, T. (1997). A probabilistic analysis of the Rocchio algorithm with TFIDF for text categorization. In International Conference on Machine Learning (ICML)
Koller, D. & Sahami, M. (1997). Hierarchically classifying documents using very few words, in ‘Proc. of the 14th Int. Conf. on Machine Learning. Nashville, US, pp. 170–178.
Labrou, Y. & Finin, T. W. (1999). Yahoo! as an ontology: Using Yahoo! categories to describe documents, in ‘Proc. of the 8th Int. Conf. on Information Knowledge Management. Kansas City, MO, pp. 180–187. DOI: https://doi.org/10.1145/319950.319976
Lewis, D. and Ringuette, M. (1994). A comparison of two learning algorithms for text categorization. In Third Annual Symposium on Document Analysis and Information Retrieval. Pp. 81-93.
McCallum, A. K. & Nigam, K. (1998). A comparison of event models for Na¨ıve Bayes text classification, in ‘Proc. of the Workshop on Text Categorization (AAAI98)’, Madison, WI, pp. 41–48.
McCallum, A. K., Rosenfeld, R., Mitchell, T. M. & Ng, A. Y. (1998). Improving text classification by shrinkage in a hierarchy of classes, in ‘Proc. of the 15th Int. Conf. on Machine Learning’, Madison, US, 359–367.
Nigam, K., McCallum, A., Thrun, S. and Mitchell, T. (1998). Learning to classify text from labeled and unlabeled documents. In Submitted to AAI-98. http://www.cs.cinn.edu/~mccallum. DOI: https://doi.org/10.21236/ADA350490
Paruchuri, H. (2017). Credit Card Fraud Detection using Machine Learning: A Systematic Literature Review. ABC Journal of Advanced Research, 6(2), 113-120. https://doi.org/10.18034/abcjar.v6i2.547 DOI: https://doi.org/10.18034/abcjar.v6i2.547
Paruchuri, H. (2018). AI Health Check Monitoring and Managing Content Up and Data in CMS World. Malaysian Journal of Medical and Biological Research, 5(2), 141-146. https://doi.org/10.18034/mjmbr.v5i2.554 DOI: https://doi.org/10.18034/mjmbr.v5i2.554
Paruchuri, H., & Asadullah, A. (2018). The Effect of Emotional Intelligence on the Diversity Climate and Innovation Capabilities. Asia Pacific Journal of Energy and Environment, 5(2), 91-96. https://doi.org/10.18034/apjee.v5i2.561 DOI: https://doi.org/10.18034/apjee.v5i2.561
Salton, G. (1991). Developments in automatic text retrieval. Science, 253: 974-979. DOI: https://doi.org/10.1126/science.253.5023.974
Sasaki, M. & Kita, K. (1998). Rule-based text categorization using hierarchical categories, in ‘Proc. of the IEEE Int. Conf. on Systems, Man, and Cybernetics. La Jolla, US, 2827–2830.
Stein, C. (1955). Inadmissibility of the usual estimator for the mean of a multivariate normal distribution. In Proceedings of the Third Berkeley Symposium on Mathematical Statistics and Probability 1. Pp. 197-206. University of California Press. DOI: https://doi.org/10.1525/9780520313880-018
Toutanova, K., Chen, F., Popat, K. & Hofmann, T. (2001). Text classification in a hierarchical mixture model for small training sets, in ‘Proc. of the 10th Int. Conf. on Information and Knowledge Management. Atlanta, USA, pp. 105–112. DOI: https://doi.org/10.1145/502585.502604
Vadlamudi, S. (2015). Enabling Trustworthiness in Artificial Intelligence - A Detailed Discussion. Engineering International, 3(2), 105-114. https://doi.org/10.18034/ei.v3i2.519 DOI: https://doi.org/10.18034/ei.v3i2.519
Vadlamudi, S. (2016). What Impact does Internet of Things have on Project Management in Project based Firms?. Asian Business Review, 6(3), 179-186. https://doi.org/10.18034/abr.v6i3.520 DOI: https://doi.org/10.18034/abr.v6i3.520
Vadlamudi, S. (2017). Stock Market Prediction using Machine Learning: A Systematic Literature Review. American Journal of Trade and Policy, 4(3), 123-128. https://doi.org/10.18034/ajtp.v4i3.521 DOI: https://doi.org/10.18034/ajtp.v4i3.521
Vadlamudi, S. (2018). Agri-Food System and Artificial Intelligence: Reconsidering Imperishability. Asian Journal of Applied Science and Engineering, 7(1), 33-42. Retrieved from https://journals.abc.us.org/index.php/ajase/article/view/1192
Wang, K., Zhou, S. & He, Y. (2001). Hierarchical classification of real life documents, in ‘Proc. of the 1st SIAM Int. Conf. on Data Mining. Chicago, USA. DOI: https://doi.org/10.1137/1.9781611972719.22
Wang, K., Zhou, S. & Liew, S. C. (1999). Building hierarchical classifiers using class proximity, in ‘Proc. of the 25th Int. Conf. on Very Large Data Bases. Edinburgh, UK, 363–374.
Yang, Y. (1999). An evaluation of statistical approaches to text categorization. Information Retrieval, 1(1-2), 69–90. DOI: https://doi.org/10.1023/A:1009982220290
--0--
Downloads
Published
Issue
Section
License
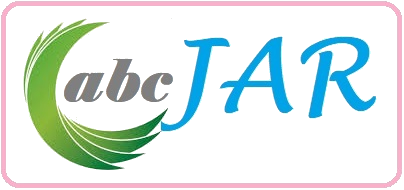